Customer Lifetime Revenue and Purchase Likelihood
Customer lifetime revenue and purchase likelihood are predictive insights about customers who have made at least one purchase in your online store. While both tools forecast future buying behavior, how you think about them from a marketing perspective may differ.
This article explains how CLR and purchase likelihood work and offers suggestions for using them in marketing engagement, time-based ROI evaluation of marketing channels, and as high-level KPIs.
Chord's Customer Lifetime Revenue (CLR) and purchase likelihood are advanced segmentation tools that use historical data to predict a customer's likely purchase behavior in the future.
CLR and purchase likelihood are commonly applied to segmentation for re-engagement actions, better understanding and targeting of new customer acquisition, and—at a high level—can be used as a nearly real-time metric for predicting a customer's long-term relationship with a brand.
At the core of predictive revenue analysis is the tried-and-true marketing concept of RFM.
RFM is a simple measure of a customer's recency, frequency, and monetary statistics. Generally, RFM describes the metrics businesses benefit from optimizing, and CLR is a bundle of tools used to measure progress on those optimizations. These metrics and how they can be used with CLR tools will be covered in more detail below.
CLR and purchase likelihood are available to Chord customers who have at least:
- 2000 completed transactions and nine months of transaction history
- 90 cumulative days containing transactions
- 500 completed transactions from the last 90 days and
- transactions completed in the past 30 days
RFM is included in Chord’s predictive revenue toolbox and can be combined with CLR and purchase likelihood.
CLR refers to a customer's total revenue for a business throughout their relationship. This includes all the customer's historical purchases and their predicted future purchases.
Purchase likelihood refers to the probability that a customer will make another purchase from a business in the future. A high purchase likelihood indicates that a customer is likelier to become a loyal, repeat customer. In contrast, a low purchase likelihood suggests that a customer may be less likely to return and make additional purchases.
RFM are staples of predictive marketing segmentation and are core building blocks of CLR and purchase likelihood. They are baked into Chord’s revenue customer segmentation toolbox. These tools are related: RFM represents summary statistics of historical data, while CLR and purchase likelihood model historical data to make future predictions.
RFM consists of the following:
- Recency: how recently a customer has made a purchase
- Frequency: how often a customer has purchased
- Monetary Value: how much the customer has spent
Together these estimates can be combined to create segmentation models.
Chord’s revenue segmentation toolbox includes simplified buckets for generating segments. The categories are broken down into 5 buckets where 1 is always the “best” or most valuable, and 5 is the lowest. For instance, Recency bucket 1 represents customers that have purchased most recently, and bucket 5 are those that purchased the least recently. Frequency bucket 1 is those customers who buy the most frequently, and 5 is the least frequent. And Monetary value bucket 1 represents the highest total spenders while bucket 5 customers are the lowest.
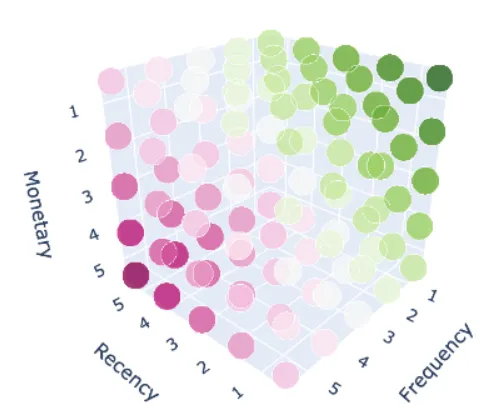
Every customer is categorized into buckets 1-5 for all three categories. The graph above shows groups of customers that fall into each R-F-M bucket. We refer to them in the order R-F-M, so a “1-1-1” is a customer who purchased most recently, is in your store’s highest frequency, and also in your store’s highest monetary spend compared to all customers.
1-1-1 (top right) are star customers: they have spent the most with the most transactions and have engaged recently
5-5-5 (bottom left) are at-risk customers: they have spent in the lowest 20% of spenders, only made one purchase, and have not purchased in a long time (relative to the store’s age)
In between these two extremes are groups of customers on a gradation of value and engagement. The broad goal of the RFM matrix is to push customers to the upper right of the graph, as represented above.
A store’s 1-1-1’s and 5-5-5’s for R-F-M are the most valuable and least valuable customers. Still, the most effective application of R-F-M usually comes with a combination of categories.
For example, a 1-3-3 is a very recent customer who spent in the store’s middle statistical range and spent an average amount of money.
You might label this customer as a “potential rising star” and plan their marketing engagements accordingly. A 2-1-2 is a high-frequency customer that could be at risk of slipping into an inactive state. You might label this customer as a “high impact potential risk” and tweak their engagement touches accordingly. The core of R-F-M is that these different groups represent different levels of engagement and realized value.
To determine CLR and purchase likelihood, a store’s historical data is modeled and then model parameters are applied to individual users. The model considers classic RFM attributes as well as customer segmentation demographics. Some attributes used in the model include:
- Customer age
- How recently the customer has purchased
- How often the customer has purchased
- Total amount the customer has spent
- Customer engagement in promotional events
A customer’s purchase likelihood and corresponding CLR can change based on their attributes and activity. For instance, if a customer makes a new purchase then their CLR will increase both for their historical value and for what we expect them to spend in the future. New predictions are updated weekly and include both new customers over that time period and updated estimates of existing customers.
Segmentation actions from predictive data should be used at a grouping level. For example, you may want to target all customers who have a predicted repurchase percentage between 65% and 75%. This segment can be further spliced by user attributes, such as geographic location or demographics, and can be combined with RFM analysis to generate detailed segments of your data.
Because an idea of future behavior is built into the statistics, one of the most powerful levers of prediction analysis is that it can be used for evaluating the outcome of retargeting events. For example, imagine a company used the purchase likelihood slice mentioned above (repurchase likelihood between 65% and 75%), held out a portion of the segment to calibrate evaluation, and then ran a retargeting campaign for those customers. At the end of the campaign, they can test whether the initial segments stayed true to their predictions—they were expected to repurchase between 65% and 75%—and then compare those with the group that got the campaign (see caveats below). The delta between these two groups is the efficiency measure of the campaign. Relatedly, the delta between the CLR of the two groups at the end of the campaign is the increase in total expected revenue and can be compared to the cost of the campaign to calculate ROI.
Evaluation of the accuracy of the predictive models is achieved using an out of sample strategy where we hold out a certain portion of the data, make predictions using the training data, and then evaluate how accurate our models were in predicting the evaluation period.
For example, a customer level view of our training and evaluation data is in the graph below.
Model Evaluation: Customer First and Subsequent Purchases
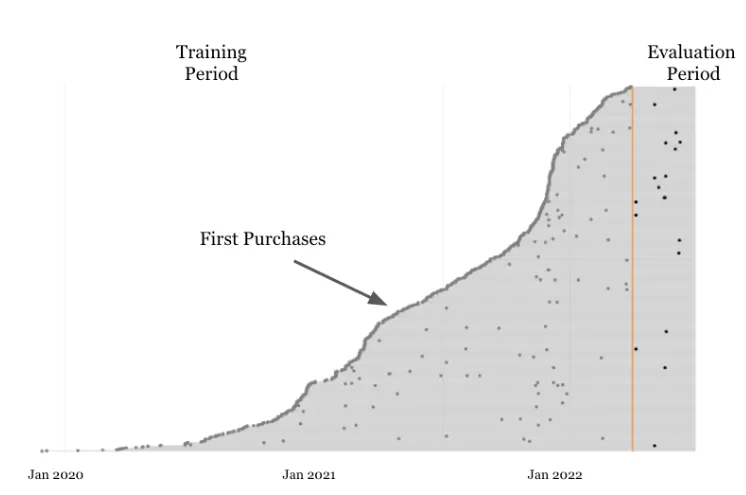
The graph above represents how we use historical data to train models to predict the future purchases.
It is important to note that our models—like all statistical models—cannot perfectly predict the future. Many variables are beyond the control of any store, e.g., macroeconomic influences and ever-changing customer preferences. There are also variables within a store’s control—changes in marketing spend, promotion, price changes, product catalog changes—that Chord has no knowledge of and, therefore, cannot account for in its models.
However, our models use stores’ historical variation to make predictions capturing trends in customer behavior. Batches run at regular intervals to keep predictions fresh and leverage as much data as we have access to deliver the most accurate predictions possible.
To find these new features:
Navigate to chord.looker.com and sign in
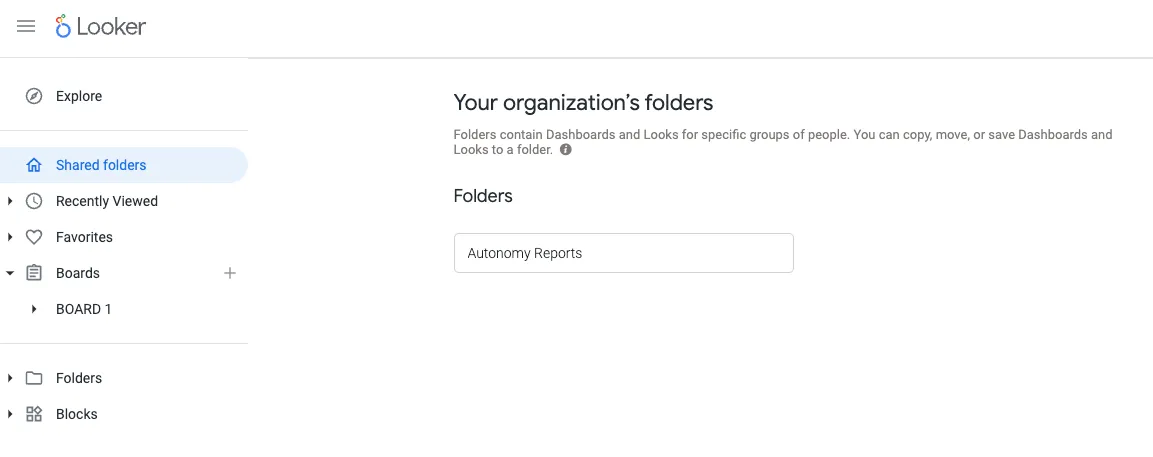
Click into the Autonomy Reports (or Performance Reports) folder
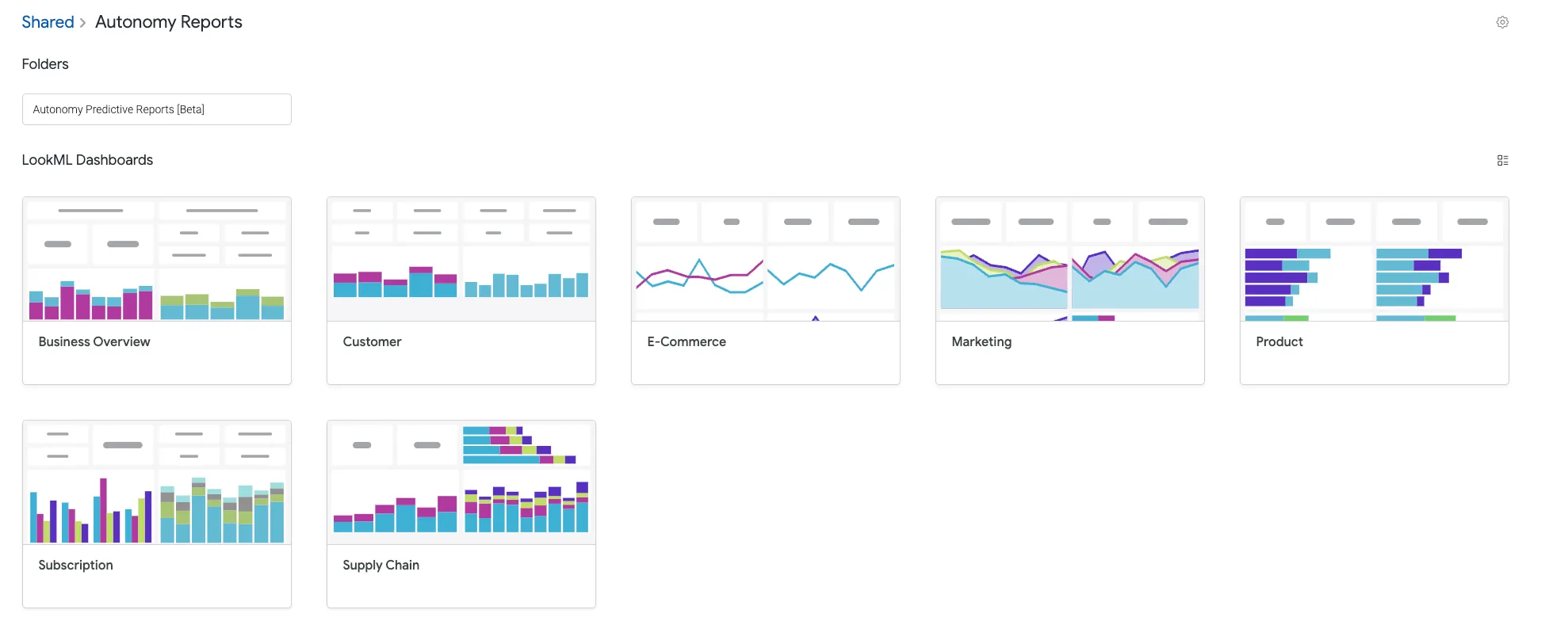
Click into the Autonomy Predictive Reports [Beta] folder (or the Performance Predictive Reports [Beta] folder) to access the dashboard tile below, which includes the predictive tiles
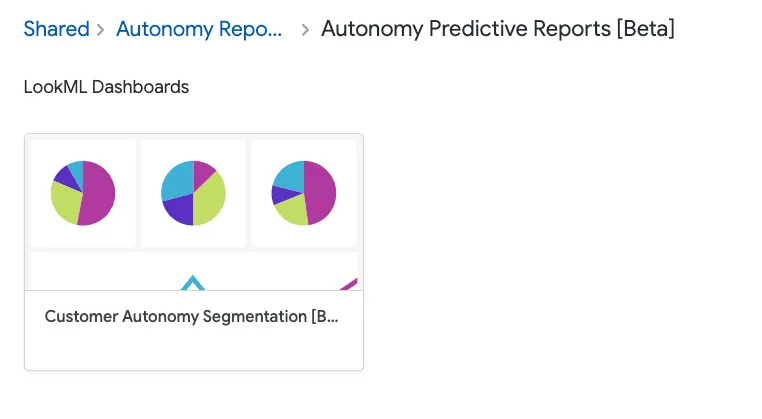
To open the Users Explore including the new predictive fields, click the Explore link at the top of the menu on the left side of the screen
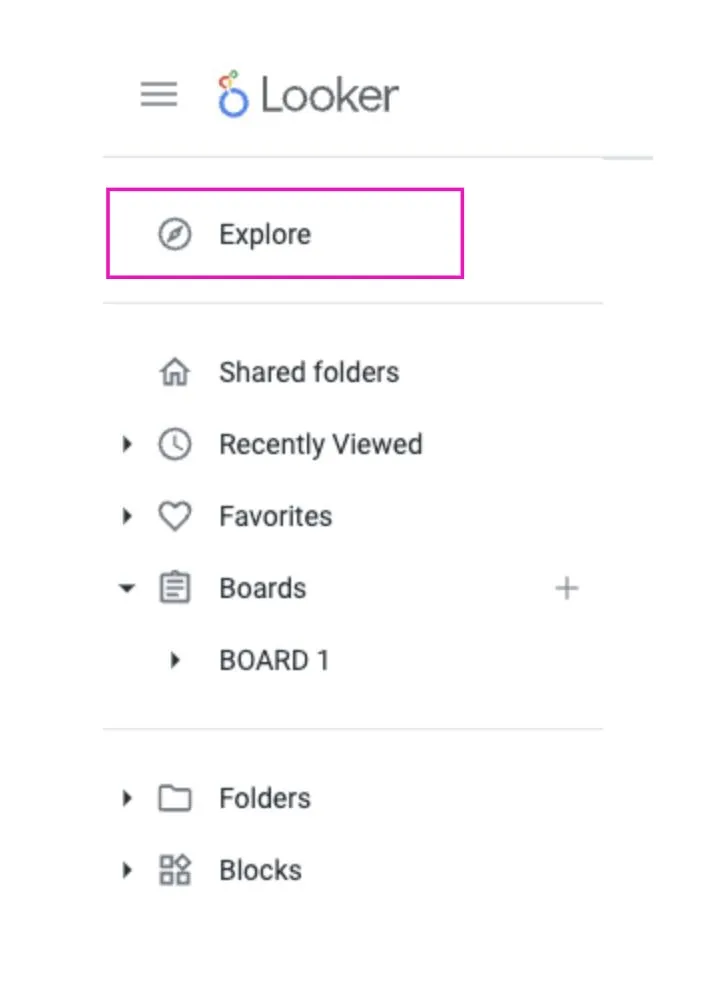
Click to select and open the Users Explore
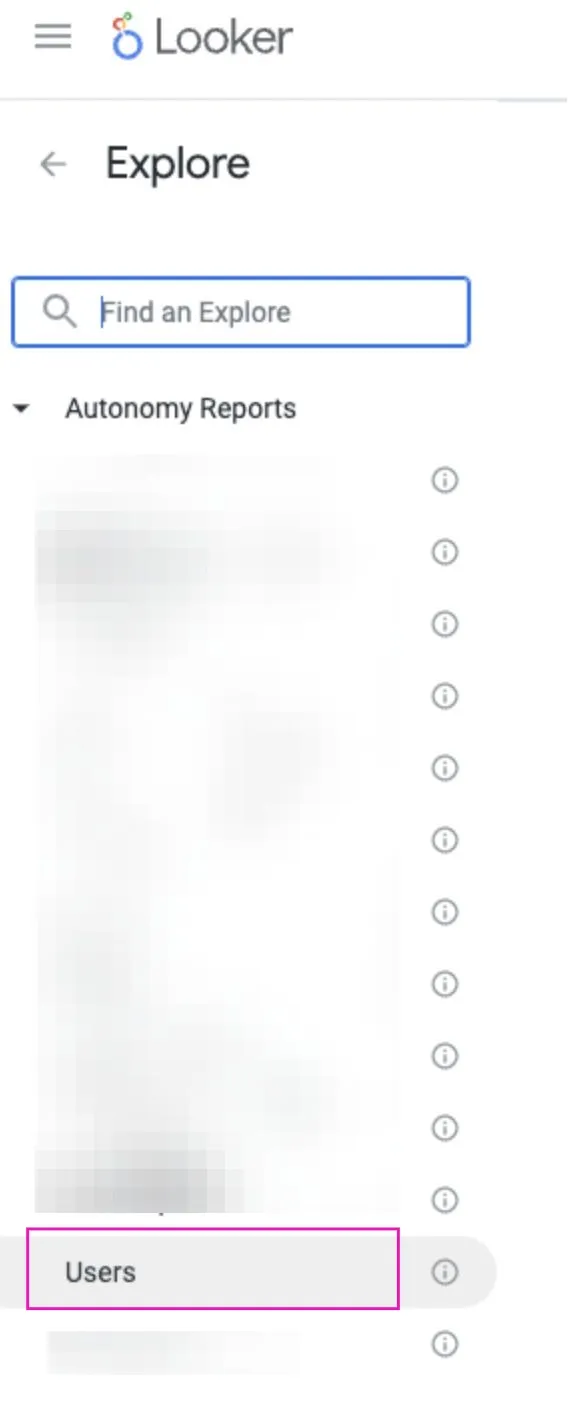
Within the Users Explore, click down arrows or group names on the left menu to display the new predictive fields that can be added to the Explore as fields, filters, or pivot points
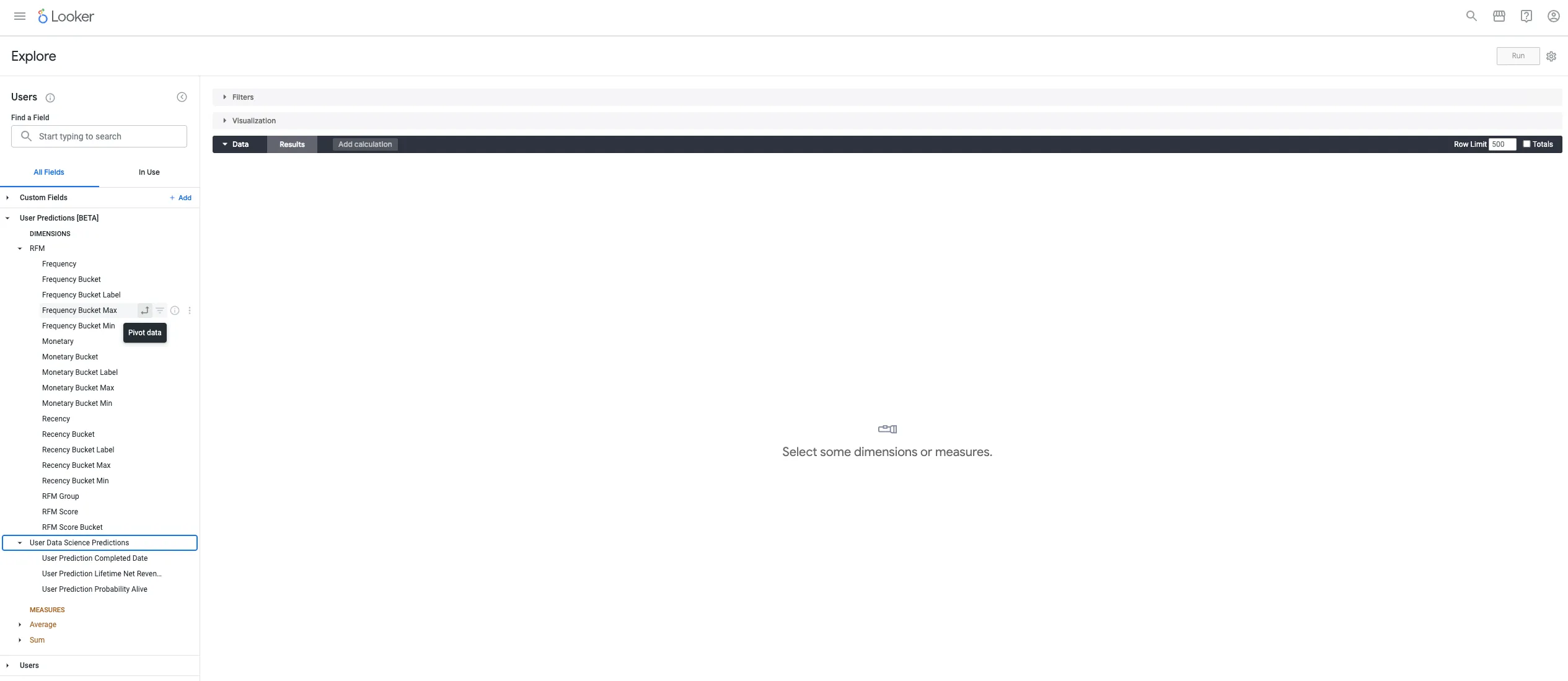
Q: How often is this refreshed / how up to date are the predictions?
A: Predictions are updated weekly and include both new customers over that time period and updated estimates of existing customers
Q: How far out are the predictions cast?
A: Two years
Q: Is the predicted revenue gross or net?
A: Our predictions use net revenue.
Q: Can I filter out / normalize customer lifetime revenue? If we had an initial launch sale or a big holiday promotion, I’d like to exclude those transactions and users from the data set because they’ll skew the averages.
A: There are no easy controls available for this right now, but Looker’s baked-in filters allow for lots of customization by filtering on date, repeat purchase vs. first-time purchase, promo code applied, etc.
Q: Is there a glossary for reference?
A: Check out our CLR and RFM data table legend