Revenue Forecasts
Time series forecasts generate day-level forecasts for input series. Forecasts are generated a daily level in an ensemble time series forecasting system that blends weights via out-of-sample accuracy. Series are forecasted for the next 24 months. While series are generated on a daily level, they can be aggregated to the weekly or monthly level by aggregating over various time periods using the date dimension. Confidence intervals are based on variability of input series and do not include large system variability, such as macroeconomic changes.
Note: Forecasts provided by Chord are best guess predictions based on the input data, are provided “as is” for analytics purposes, and do not constitute any guarantee explicit or implied of actualization of forecasts.
Current forecasted series:
- Net Revenue: total daily revenue for all customers.
- Reoccurring Revenue: revenue generated by non-first purchase or "repeat" customers.
- First-Time Purchase Revenue: revenue from first-time purchase customers.
- Number of First-Time Customers: total number of new customers.
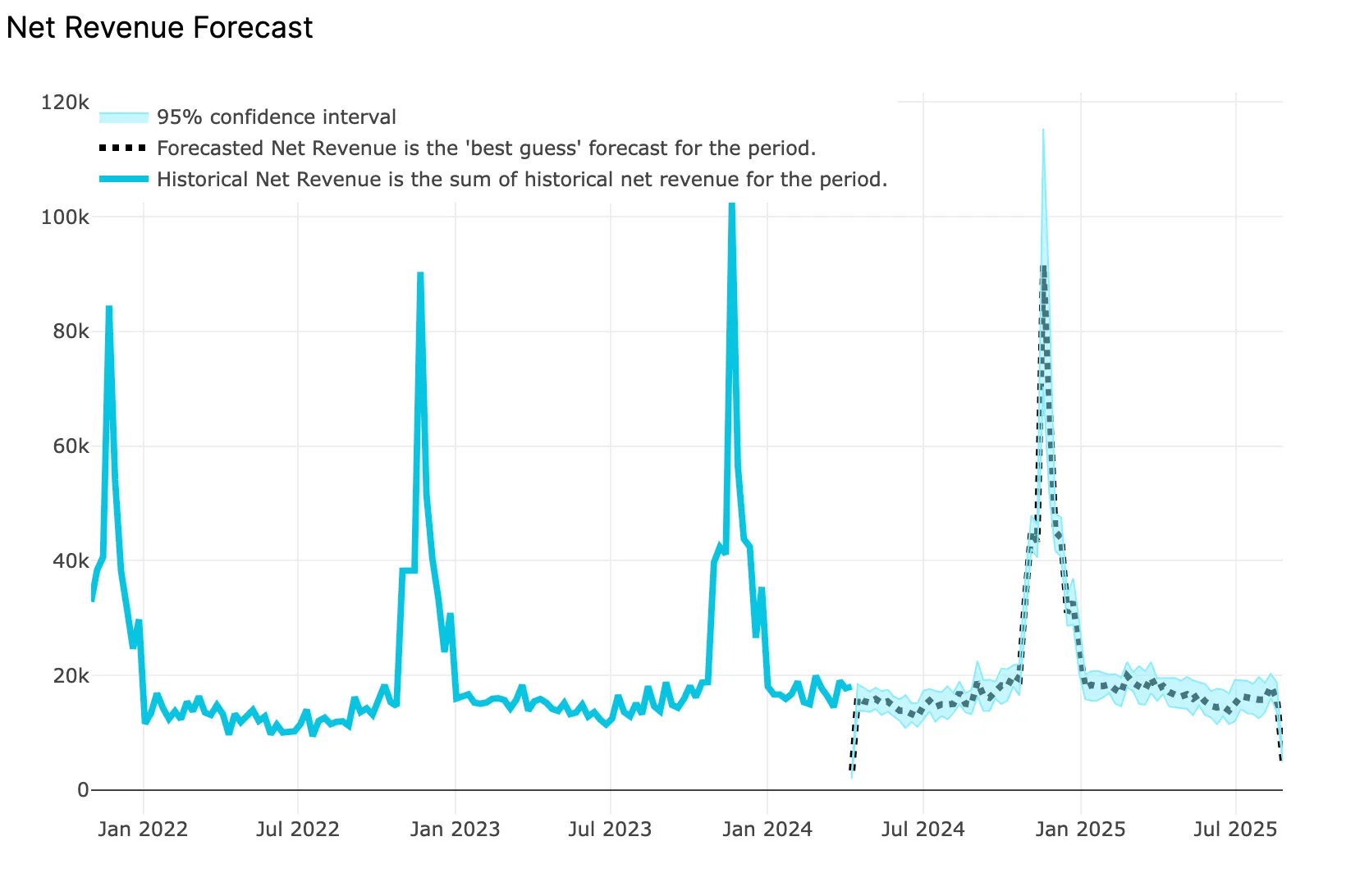
Time series models are generated using a range of time series modeling procedures, including ARIMA, Regression, and Theta models, and control for a range of seasonality indices and special events, such as holidays and common retailing events (Black Friday). These models are evaluated using out-of-sample (or “holdout”) periods that folded across short- and long- range holdouts into “best guess” forecasts. The variation for our confidence intervals is generated from the variation in the ranges of input ensemble models.
Our models do not include exogenous variation outside of the series itself. This means that we are forecasting that series in isolation of outside influences. These exogenous influences include the macroeconomy and any other factors that could impact the series, including changes in a store’s product assortment or pricing. Similar to nearly all-time series forecasting models, these models should be interpreted as “all else equal” analyses; that is, everything outside the variation on this single series stays the same; these are the “best guess” estimates for the input series.
Accuracy is calculated via out-of-sample forecasts. 30, 60, and 90 day forecast are generated by holding the hold-out time periods from the data and forecasting from the new end date. The forecast of these models is compared with the actuals that happened during the hold-out period, along with the average mean absolute percentage error (MAPE).
Forecasts are generated daily for the next two years.
Tenant stores are eligible if they have two years of history and over 1k transactions.